Data Engineering Services
Improve efficiency of data pipelines on cloud and operationalize AI platformsHome / What we do / Data Engineering
Build powerful data platforms that deliver faster insights
Analysts and data scientists of large enterprises usually spend over 70% of their time in data processing rather than on analysis and insights generation. Sigmoid’s data engineering services aim to solve data-related challenges by building efficient data pipelines that modernize platforms and enable rapid AI adoption. We help organize and manage your data better, generate faster insights, build predictive systems,and effectively collaborate with the data science teams to extract the highest ROI from your data investments
Data engineering services to strengthen your data and analytics initiatives

Data Pipelines
Leverage our data warehousing expertise to build efficient data pipelines, enhance query performance, and generate faster insights. Automate data ingestion from diverse sources with our data connectors and low-code, no-code frameworks.

ML Engineering
Strength your ML model lifecycle management and accelerate time to business value for AI projects. Move from proof-of-concept to production by deploying robust ML models that can effectively scale across brands and geographies.

Cloud Transformation
Improve business agility and minimize infrastructure costs with cloud transformation. Our experts assess and architect the ideal cloud setup for your business and help in seamless data migration without risking production SLA and quality.

DataOps
Effective enterprise data management and governance to reduce downtime and mitigate data risks. Leverage our proven DataOps services to experience high availability, CI-CD pipelines, testing, and monitoring of enterprise data operations.
How data engineering amplifies business value of advanced analytics
Well-defined data engineering processes create a robust foundation for consistently delivering insights at scale. Read our whitepaper to find out how you can build an efficient data engineering team and maximize business value.
Download whitepaper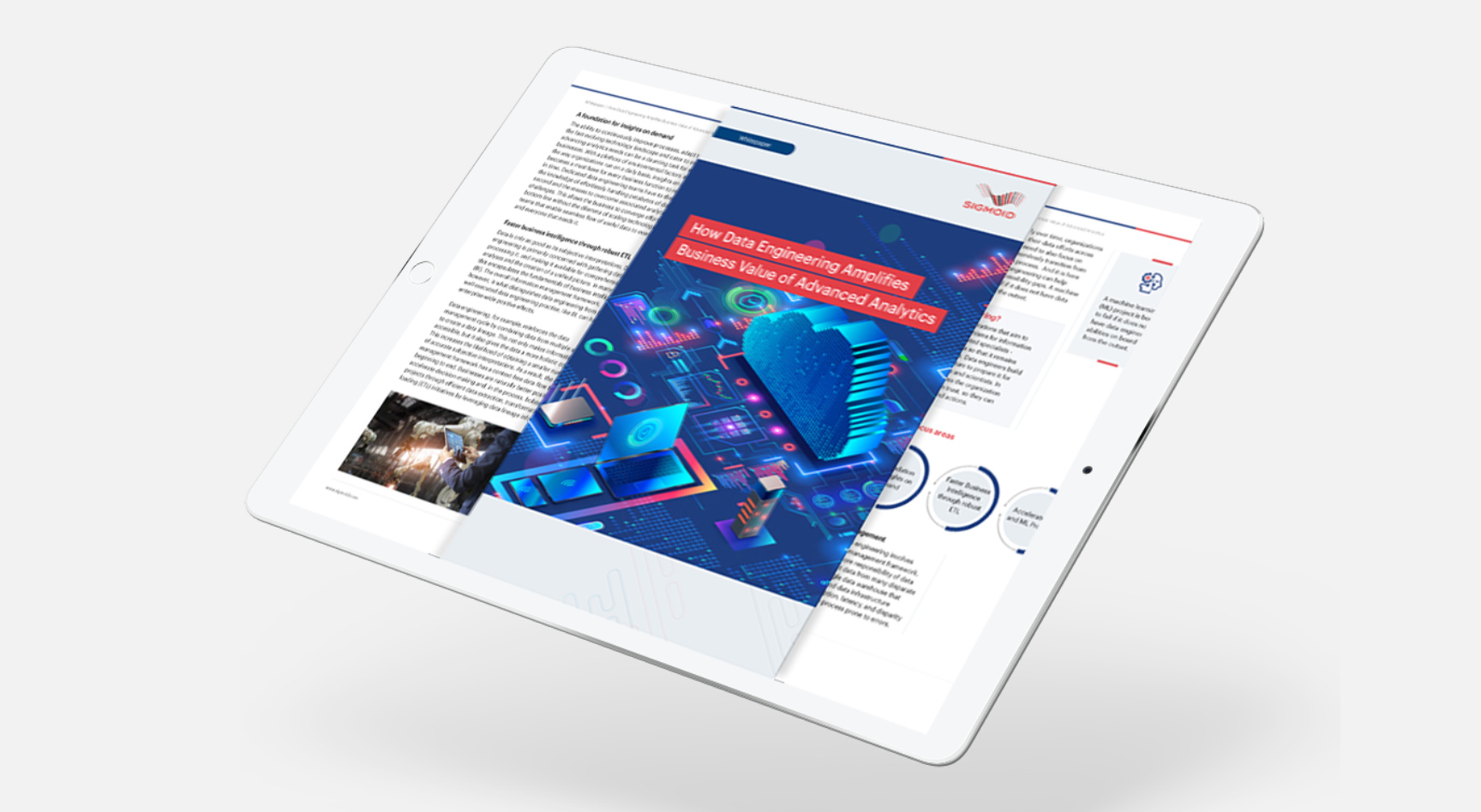
Customer success stories
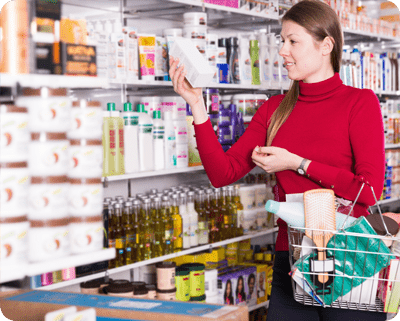
Automated data ingestion from 10+ retailers with data lake for CPG firm
Built a data lake to capture and automate diverse data from 10+ retailers and ecommerce sites, enabling real-time insights into sales trends for a CPG company.
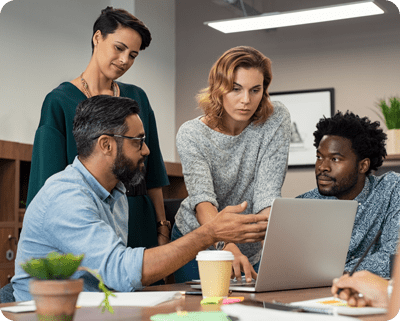
4x faster data pipelines to enhance regulatory compliance for F100 bank
Efficiently streamlined 100 MN rows of asset class and market data daily to reduce processing time and minimize false alerts for a top-3 global investment bank.
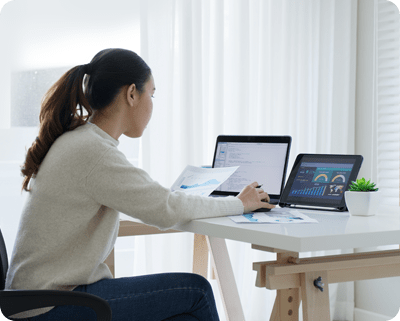
65% cost savings with efficient migration to Google Cloud for AdTech firm
Developed a Spark-based ETL framework on GCP to optimize data infrastructure landscape and deliver over $2.5 MN annual cost savings for an AdTech firm.
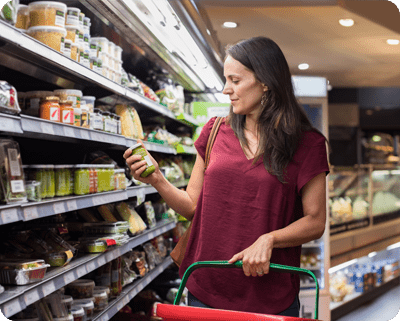
MLOps delivers 90% improvement in model runtime for FMCG company
MLOps solution helped a CPG client scale their pricing and promotions ML models across geographies and reduce the model runtime from 8 days to just 14 hours.
Data engineer at Sigmoid
- Experienced in different cloud platforms, open-source technologies and full data technology stack
- Extensive certification across leading data engineering tech stack such as AWS, GCP, Azure, Snowflake, Matillion, Dataiku, and Databricks
- An investment of $10,000 per data engineer in technology training across six months
- Cross-skilled and communicates effectively with data scientists to deliver complex ML projects
- Leverages data engineering best practices and Sigmoid’s agile framework for data
Explore our other data and analytics offerings

Data strategy
Build a robust analytics roadmap and modernize your data fabric for driving business transformation.

Data science
Get faster actionable business insights using data science, visualization, and AI for a high success rate on your analytics initiatives.

Accelerators
Leverage our pre-built analytics assets and proprietary frameworks to accelerate data-to-value for your business.