ML based image processing with Google Vision delivers deep insights into consumer buying patterns
Sigmoid developed an image analytics based solution powered by deep learning to gain insights into consumer buying patterns, product preferences, and brand penetration. By analyzing product images from consumers' shelves, the consumer insights team was able to identify untapped segments, optimize marketing spends and validate market share.
Business Challenge
An American F100 consumer products manufacturer wanted to enrich the existing consumer dataset in order to gain deeper insight into their sentiment, behaviour, and purchase actions. This analysis would help them understand how their products fit into the lives of their consumers and empower advanced segmentation and personalization strategies. Relooking at their brand penetration vs other competitive brands in the personal care segment validated their ground share, while comparing the data from other market research agencies. This exercise was crucial as it would directly impact their marketing initiatives, digital orchestration, campaign strategy, and resource allocation.
Sigmoid Solution
Sigmoid created an app-ready solution using Google Vision API to identify products and brands in images. Consumer shelf images were uploaded into the OCR pipeline, where different image enhancement and filtering techniques were applied to reduce blurs, improve gradients, contrast & brightness adjustments, clarity, etc. The Vision API recognized product and brand information, including text, logos, descriptions, colours, and objects, using predefined categories. Advanced machine learning algorithms annotated each image object with its product class information. Custom NLP algorithms were programmed to eliminate duplication and remove non-relevant products. Probabilistic matching techniques helped accurately detect all the brands in the images and map products to their respective brands.
Business Impact
Sigmoid’s self-learning solution enabled the client to deeply understand their brand penetration compared to competition and consumer behavior. It would help them categorize customer segments with low and high brand penetration and devise personalized marketing strategies and reallocate the marketing budget.
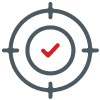
98% accuracy
achieved in identifying beauty products and associated brands
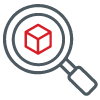
96% precision
in detecting in-house brands and competing brands

Improved
consumer intelligence to devise personalized marketing campaigns