Transforming trade surveillance analytics with cloud data management
Reading Time: 5 minutes
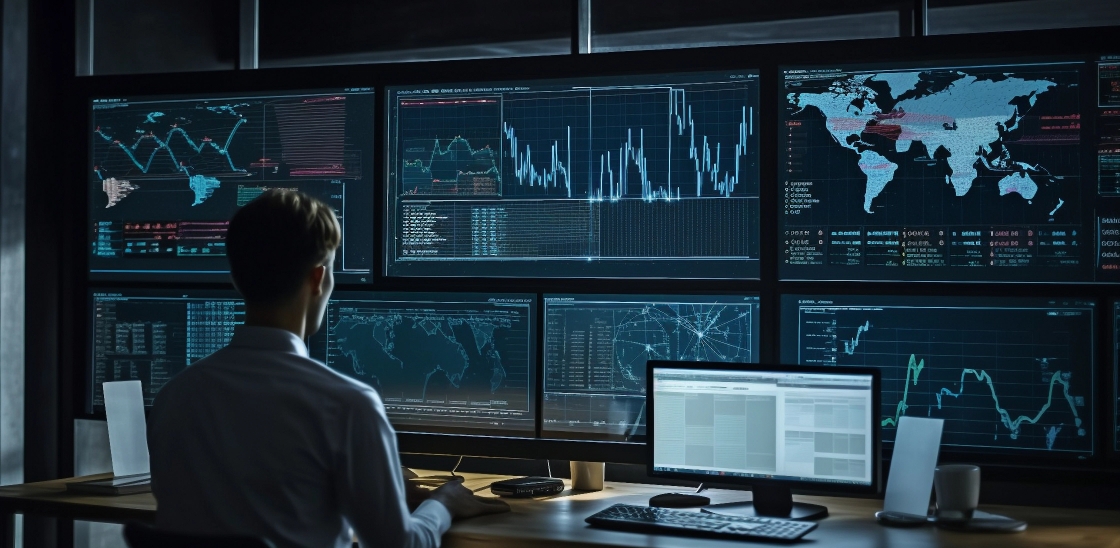
The proliferation of diverse communication channels across devices and applications has significantly amplified the complexity of maintaining robust trade surveillance. As possible perpetrators innovate novel ways to exchange information, trade surveillance has become increasingly complex. A comprehensive study involving senior executives from banks, brokerages, and proprietary trading firms revealed a consensus, with 94% acknowledging a surge in the complexity of trade surveillance over the past three years.
This surge is a result of several factors, including the evolving regulatory rules and the emergence of diverse asset classes, such as cryptocurrencies. Adapting to these shifts and uncovering previously unnoticed patterns has become a pressing mandate for market participants.
Compliance analysts at most tier one banks review about 1000 alerts a day, over 99% of these alerts turn out to be false.
Cloud scalability and AI for market oversight
Organizations are exploring various financial data analytics approaches for faster and accurate trade surveillance. Cloud-based data management emerges as a standout solution, offering scalability, real-time processing, and ease of integration with AI/ML-based solutions.
Cloud transformation has revolutionized the way businesses handle data, and trade surveillance analytics is no exception. The ascent of machine learning relies on efficient cloud-based data management for real-time market insights. This dynamic synergy empowers efficient processing and analysis, enabling businesses to extract valuable insights and enhance decision-making.
Key components of a cloud-based trade surveillance system
The dynamic market conditions and heightened regulatory requirements necessitate an immediate transformation in trade surveillance systems. Cloud scalability and AI integration play pivotal roles in enhancing each stage of a cloud-based trade surveillance system.
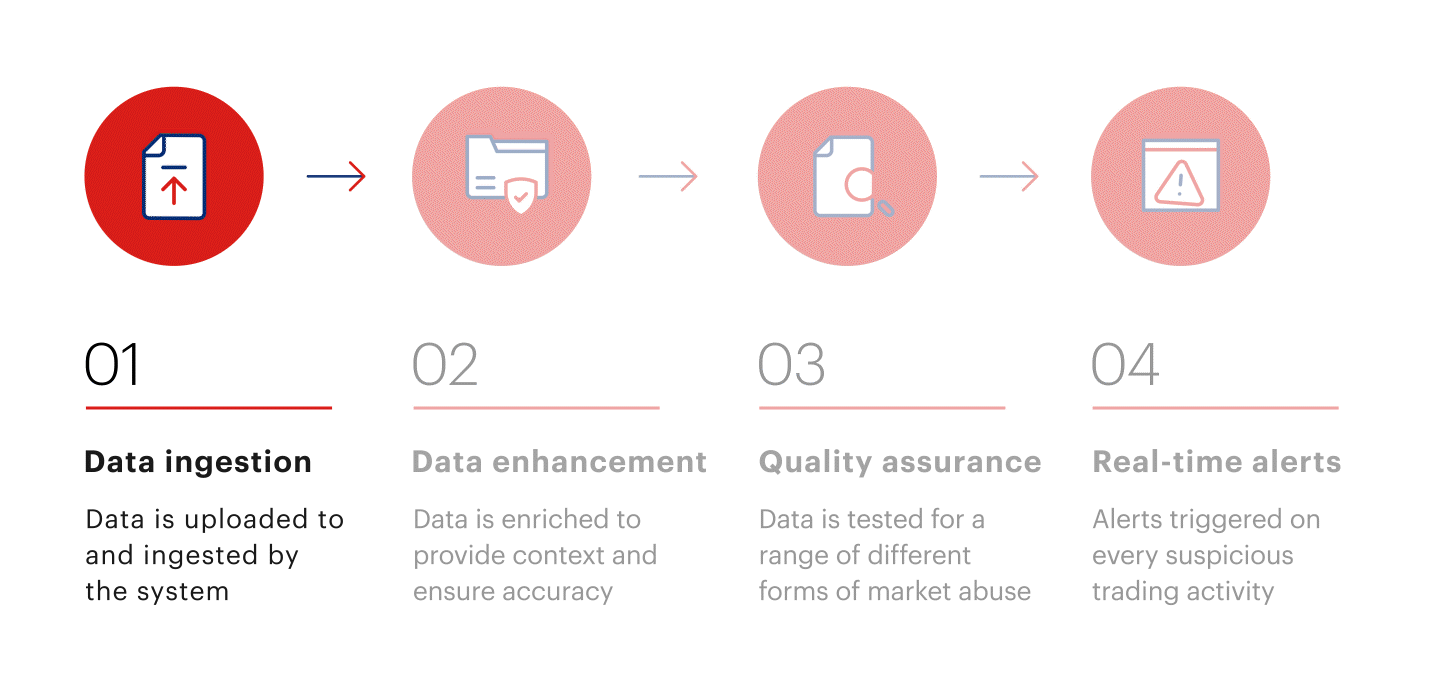
- Data ingestion: A cloud-centric trade surveillance system streamlines the process of ingesting large volumes of trade data into cloud storage solutions like Amazon S3, Google Cloud Storage, or Azure Blob Storage. Leveraging cloud-native capabilities enables seamless scalability, allowing the system to efficiently handle varying data loads.
- Data enhancement: Enhances trade data by integrating it with additional contextual information. Leveraging serverless computing services such as AWS Lambda, Google Cloud Functions, or Azure Functions, this component automates the enrichment process. By leveraging cloud-native AI/ML models, the system gains the ability to contextualize and enhance data dynamically. Cloud scalability ensures rapid processing of enriched data, while integration with cloud-native databases and analytics services facilitates real-time enrichment.
- Quality assurance: System testing ensures the robustness and accuracy of surveillance algorithms and models. Cloud-based machine learning platforms such as Amazon SageMaker, Google Cloud AI Platform, or Azure Machine Learning enable efficient testing at scale. Cloud resources allow parallel testing of multiple scenarios, ensuring a thorough evaluation of system performance.
- Real-time alerts: Create actionable alerts based on predefined rules and machine learning models. Leveraging cloud-native analytics services like Amazon Kinesis, Google Cloud Pub/Sub, or Azure Stream Analytics, this component enables real-time processing of trade data, promptly identifying anomalies and potential risks. Cloud scalability ensures the responsiveness of alert generation, while notification services facilitate timely communication of alerts to relevant stakeholders.
Four data obstacles to overcome in trade surveillance
Trade surveillance analytics play a pivotal role in ensuring the integrity and compliance of financial markets. However, several challenges must be addressed to effectively manage and analyze trade data. Here are the key data challenges faced by enterprises looking to enhance trade surveillance analytics:
- Data volume and processing speed:
The financial services financial services industry is characterized by vast amounts of trade data generated in real time. Managing and processing this large volume of data poses a significant challenge. Financial institutions need robust infrastructure and cutting-edge technologies to handle the sheer scale of trade data while ensuring timely processing. This challenge is amplified by the need for real-time insights to identify potential market manipulations and illicit activities promptly. - Integration of diverse data sources:
Comprehensive trade surveillance requires the integration of diverse data sources. This is usually data from market feeds, news updates, risk management systems, regulatory filings, and more. Achieving a holistic view of market conditions demands seamless integration and synchronization of these disparate data streams. Financial institutions must navigate the complexities of diverse data formats and structures to create a unified and comprehensive analytical framework. - Machine learning model deployment:
Deploying and managing machine learning models is a critical aspect of trade surveillance analytics. These models are essential for predictive analytics to identify potential market anomalies and suspicious activities. Financial institutions face challenges in selecting, training, and deploying machine learning algorithms that align with the dynamic nature of financial markets. Managing model drift, and eManaging model drift, and ensuring the accuracy and reliability of these models is paramount for effective trade surveillance. - Real-time monitoring and alerts:
Achieving real-time monitoring and timely alerts for potential market anomalies is crucial for continuous threat exposure management. Trade surveillance analytics must enable instant detection of unusual trading patterns, unauthorized transactions, and other red flags. The challenge lies in implementing systems that provide real-time insights without compromising accuracy or inundating analysts with false positives.
Case in point
Explore how Sigmoid’s innovative solutions can transform trade surveillance, as demonstrated in the case study below.
Situation | Solution | Impact |
---|---|---|
A global investment bank sought to enhance its data infrastructure to comply with regulations such as MAR and MiFID. Their goal was to establish robust data pipelines, real-time data mapping, and a customized in-house tool to ensure regulatory standards were met. | Sigmoid enhanced an existing surveillance model by identifying new scenarios and parameters to improve efficiency. We developed a detailed alerting workflow and interface, enabling tuning and iteration of the model based on compliance team requirements. The custom-built architecture allowed them to classify fraudulent transactions and implement surveillance. |
|
Conclusion
As financial markets grapple with increased complexity and regulatory demands, cloud solutions offer a scalable, flexible, and efficient pathway to navigate these challenges. The key components of a cloud-based trade surveillance system, exemplified by Sigmoid’s impactful solution, underscore the potential for substantial improvements in system response time and reduction in false alerts. Embracing cloud data management not only ensures regulatory compliance but positions financial institutions to proactively manage risks and adapt to the dynamic nature of modern markets.
References:
Featured blogs
Subscribe to get latest insights
Talk to our experts
Get the best ROI with Sigmoid’s services in data engineering and AI
Featured blogs
Talk to our experts
Get the best ROI with Sigmoid’s services in data engineering and AI