How GenAI transforms customer experiences with personalised product recommendations
Reading Time: 5 minutes
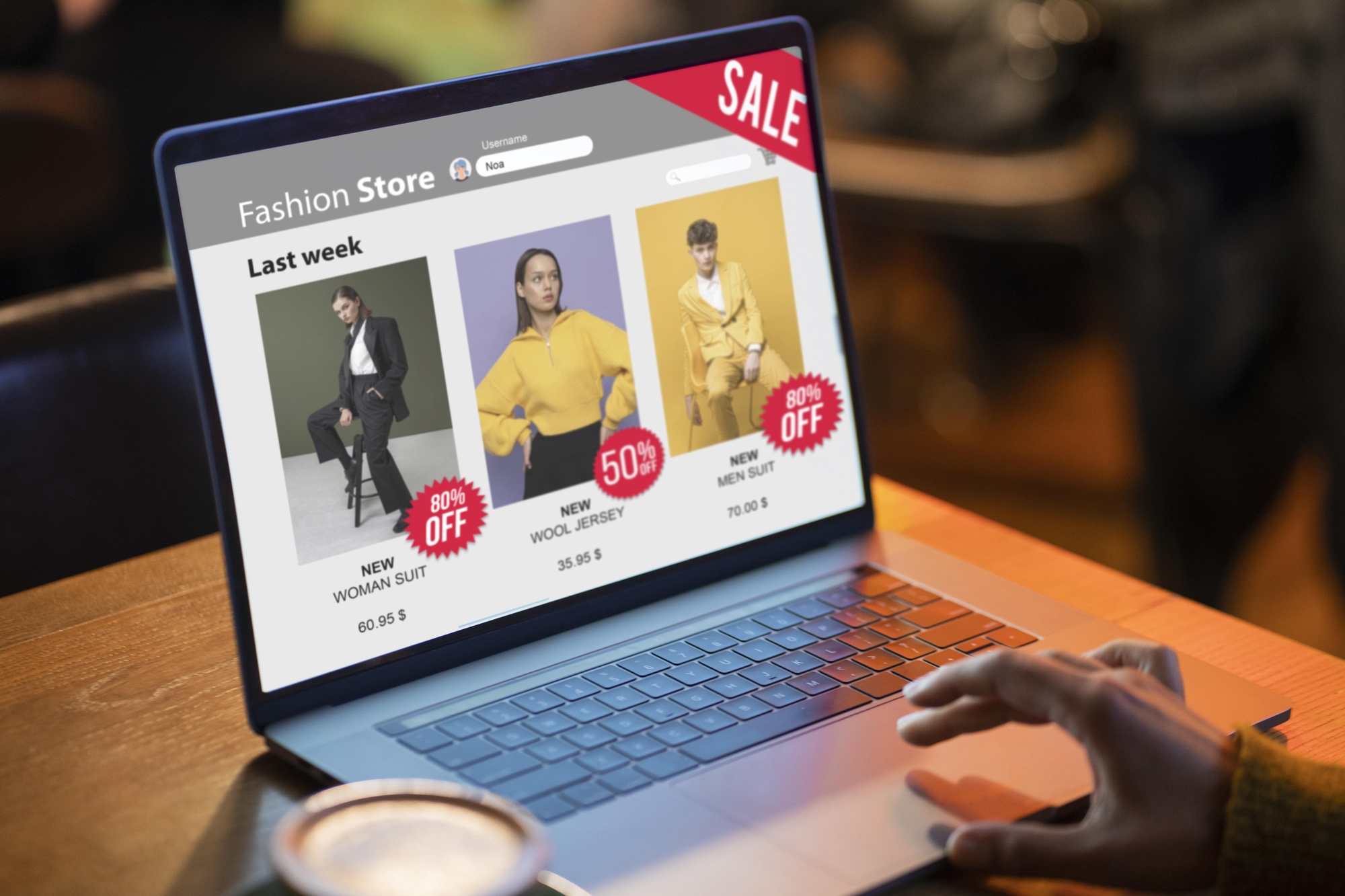
Marketing is undergoing a profound transformation, with generative AI (GenAI) pushing the boundaries of what’s possible. By enabling hyper-personalized product recommendations and accurately anticipating customer preferences, GenAI is helping to take conventional marketing approaches one notch up. Tasks that once required significant effort—like hyper-tailored product recommendations in real-time or predicting customer desires before they’re articulated—are now achieved in minutes. This shift represents more than incremental innovation; it is making customer interactions more intuitive and deeply aligned with individual expectations.
Studies show that 66% of consumers are willing to purchase new products based on GenAI recommendations, while 55% reported enhanced shopping experiences due to hyper-personalized recommendations1. These numbers not only highlight a growing consumer trust in AI-driven recommendations but also signal a paradigm shift in how shopping journeys are influenced by technology. By leveraging vast data sets and human-like creativity, GenAI is empowering businesses to create deeply personalized shopping journeys that are transforming how consumers discover, engage with, and buy products.
GenAI techniques for product recommendations
At its core, GenAI employs sophisticated techniques to enhance product recommendations including:
- Advanced collaborative filtering algorithms that leverage synthetic data generation to address cold-start problems for new users
- Natural Language Processing (NLP) for understanding nuanced customer preferences and generating contextual product descriptions
- Computer vision and image recognition for visual similarity matching and style recommendations
- Deep learning models that combine multiple data sources to create comprehensive user profiles
These systems utilize various approaches based on specific use cases such as:
- Content-based generators that analyze product attributes and user preferences
- Hybrid recommendation engines that combine collaborative filtering with generative capabilities
- Sequential recommendation models that understand shopping journey patterns
- Multi-modal systems that process text, images, and user behavior simultaneously
The integration of these techniques ensures that recommendation engines remain contextually aware to adapt, refine, and personalize suggestions effectively over time.
How GenAI reshapes customer purchase experiences
GenAI’s versatility has enabled its adoption across various facets of eCommerce and retail, including:
- Conversational commerce
- Personalized product bundles
- Virtual try-ons
- Seasonal or trend-based recommendations
- Enhanced search discovery with multi-lingual adaption
- Proactive post-purchase engagement
- Data privacy and security: Implement robust data protection measures and ensure compliance with privacy regulations while maintaining personalization effectiveness.
- AI governance: Establish clear frameworks for model monitoring, bias detection, and ethical AI usage to maintain recommendation quality and fairness.
- Technical infrastructure: Invest in scalable computing resources and efficient data processing capabilities to handle complex GenAI models and real-time recommendations.
- Continuous optimization: Regularly conduct A/B testing and model fine-tuning to improve recommendation accuracy and adapt to changing consumer preferences.
GenAI powers virtual assistants and chatbots that deliver human-like, interactive shopping journeys. For example, German online retailer Zalando has launched a new fashion assistant powered by ChatGPT to enhance the consumer experience and improve consumer perceptions of the website. For instance, when a consumer enquires about suitable attire for a spring wedding in Spain, Zalando’s fashion assistant can discern the formal nature of the event, anticipate weather conditions, and offer appropriate clothing recommendations.
GenAI facilitates contextual product bundling, which increases sales and enhances customer satisfaction by anticipating their needs. Carrefour’s online shoppers in France can choose products based on their budget and food constraints or seek new menu ideas. The ChatGPT-based chatbot can also suggest anti-waste solutions for reusing ingredients and compose baskets based on specific recipes. Nike’s recommendation engine uses GenAI to suggest complementary products based on purchase history and workout preferences for creating personalized product bundles.
Retailers are using GenAI to simulate how clothing, makeup, or accessories look on customers using uploaded images. This technology allows customers to make informed purchasing decisions, which in turn, reduces return rates. Sephora’s Virtual Artist uses GenAI to enable customers to visualize how different makeup products would look on their faces, significantly improving purchase confidence and reducing return rates.
GenAI analyzes data from social media, blogs, and online forums to predict emerging trends. Retailers can use this insight to suggest products aligned with seasonal or cultural preferences. Zara employs GenAI algorithms to analyze social media trends and customer feedback, allowing it to adjust product recommendations based on emerging fashion preferences and seasonal changes.
GenAI’s NLP capabilities make product searches smarter and more intuitive. It understands and processes vague or incomplete queries and applies context to produce precise and relevant suggestions. It allows for tailoring product suggestions to a user’s preferred or local language, ensuring accessibility for global audiences. Alibaba’s GenAI system breaks language barriers by providing accurate product recommendations and descriptions in multiple languages.
GenAI can significantly improve post-purchase engagement by delivering hyper-personalized experiences such as complementary product suggestions, loyalty reward schemes, real-time customer service through chatbots, product tutorials and care guides etc. that maintain customer interest and in turn, boost loyalty. Dyson leverages GenAI technology for its “Smart Care” program that runs for the customers post-purchase of their appliances to send personalized maintenance schedules based on usage patterns, recommend specific filters and accessories timed to the product’s lifecycle and provide customized cleaning tips and product care advice based on the user’s environment.
Considerations for implementing GenAI in marketing
Organizations must make the following provisions to successfully implement GenAI-powered recommendation systems:
Enhanced customer experiences through hyper-personalization
Sigmoid has enabled global CPG and retail brands to achieve up to 25% faster time-to-market with customer-driven solutions while transitioning sales teams from product-based to solution-based selling.
Our AI-powered solutions deliver hyper-personalized email campaigns, tailored product recommendations, dynamic content descriptions, and adaptive product messaging. Using deep learning, we analyze customer segments, behaviours, and preferences in real-time to craft custom recommendations, email content, and offers that drive engagement and conversions.
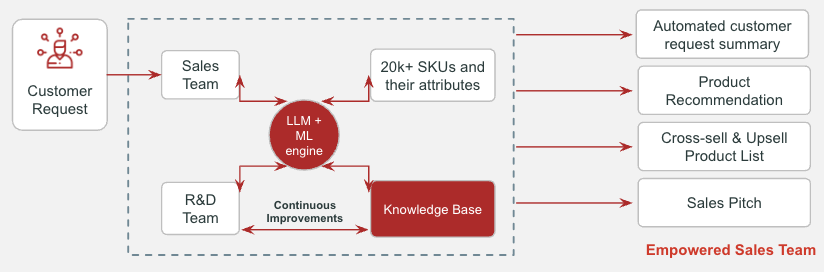
Fig 1. Sigmoid’s GenAI based recommendation framework
Our multimodal recommendation engine combines computer vision and natural language processing to recommend complementary products by analyzing both visual and textual attributes, aligning with customer preferences. We address the cold-start problem by generating synthetic user profiles that enable accurate recommendations for new users or products from the start.
The GenAI layer over our custom recommendation engine analyzes carts, purchase histories, and product relationships to create intelligent bundles and upgrade shopping paths that enhance cross-sell and upsell opportunities. This hybrid approach ensures valuable, context-aware suggestions for customers with seamless shopping experiences that boost sales outcomes.
Conclusion
Generative AI is revolutionizing product recommendation systems, setting new benchmarks for personalization and customer engagement. GenAI creates shopping experiences that are not just functional but delightful. Businesses that embrace GenAI-powered recommendations stand to gain a competitive edge in customer retention, sales growth, and market relevance. As this technology continues to evolve, it holds the promise of transforming retail and eCommerce, making every shopping journey as unique as the customer behind it.
About the Author
Bhaskar Ammu is a Senior Lead Data Scientist at Sigmoid. He brings 12+ years of experience in designing and implementing demand forecasting models, AI-driven recommendation systems and workflow automation and other advanced analytics solutions across industries like Healthcare, Retail, CPG, Manufacturing, and Advertising. He has a proven track record in driving data-driven decisions, deploying scalable machine learning models, and leveraging cloud platforms like AWS, Azure, and GCP to optimize business outcomes.
References:
Featured blogs
Talk to our experts
Get the best ROI with Sigmoid’s services in data engineering and AI