The future of demand planning with gen AI
Reading Time: 7 minutes
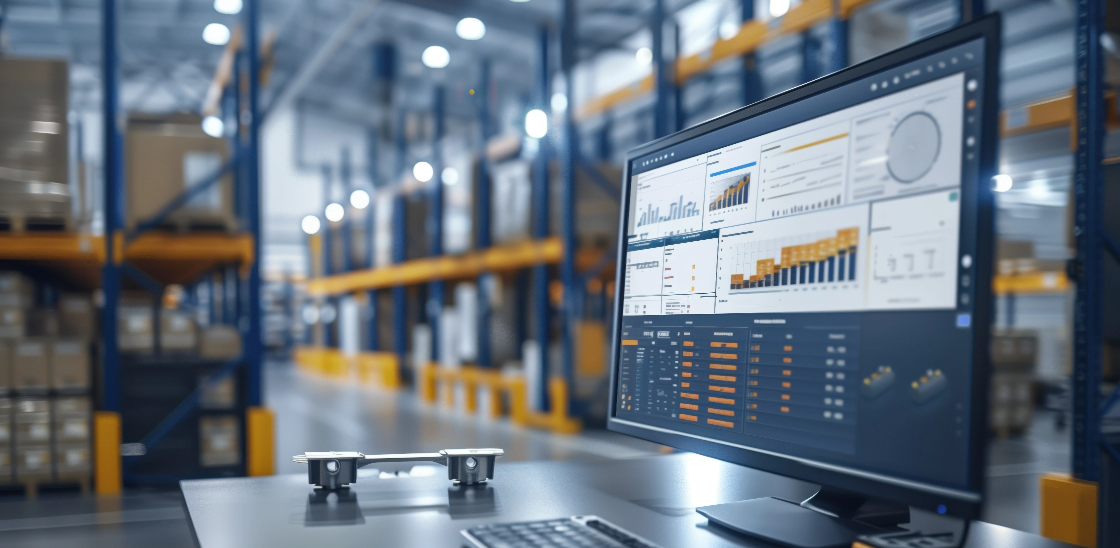
In periods of high volatility, CPGs must quickly evaluate shifting market forces and consumer demand to make informed decisions about commercial and customer investments, capacity and inventory planning, and cash allocations. These decisions must be scaled across various product categories, industries, locations, and channels served by the business. Effective demand planning and forecasting are key to building adaptable and resilient supply chains for seamless fulfillment.
This blog delves into the limitations of traditional demand planning and forecasting methods and how gen AI can enhance these processes, making enterprises more agile and responsive than ever.
Demand planning and forecasting explained
Demand planning involves predicting future sales (i.e., future demand), helping retailers and suppliers gauge the volume of customers requiring their products. The goal is to ensure the right level of inventory and align execution capacity to deliver the right products to the right customers. Techniques like scenario planning and sensitivity analysis improve forecast accuracy by creating multiple hypothetical scenarios with different assumptions to predict future demand.
Similarly, demand forecasting helps companies make informed decisions about production, inventory management, pricing, and other critical operations. It provides vital information about future customer demand, which can help in minimizing waste, reducing costs, and increasing operational efficiency. Together, demand planning and forecasting lead to better financial performance by ensuring the right products are available in the right quantities at the right place.
Why demand planning is crucial for business
Operating decisions often hinge on current market demand for a given product and a company’s ability to satisfy that demand profitably. These decisions are backed by effective demand planning that requires collaboration among key stakeholders within and outside the organization to align supply with demand.
Demand planning is a critical business practice that positively affects the bottom line, while offering additional benefits such as:
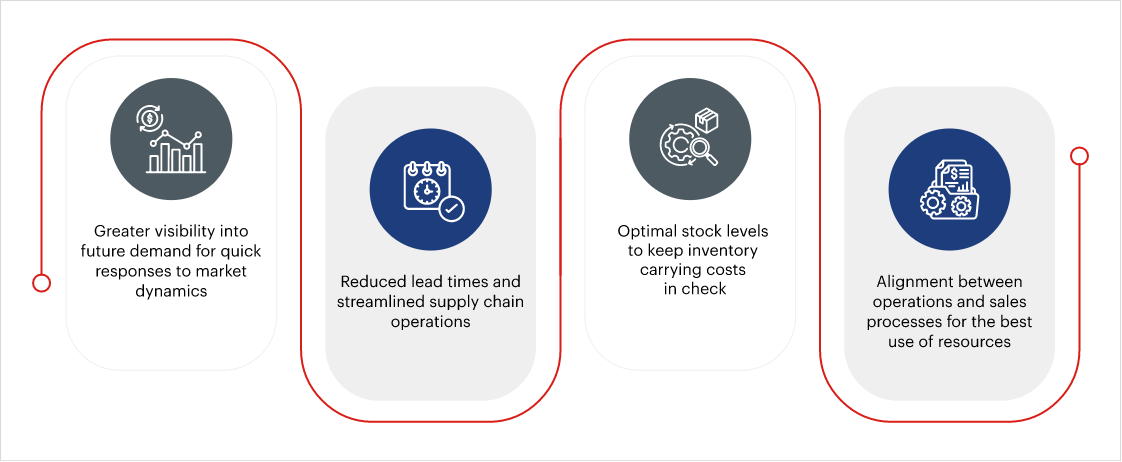
Predictive analytics for demand planning and forecasting
Currently, most businesses apply analytics techniques like statistical modeling, time series analysis, linear/multiple regression analysis or machine learning algorithms to forecast demand. The analysis typically relies on only historical data spanning 24-26 months. However, the limited scope of data and inability to account for complex market dynamics, macro-economic factors, or demand changes can lead to missed opportunities.
However, analytics-driven demand planning may fall short in the following areas:
- Identifying the source of trends or origin point of uncertainties
- Prioritize the right scenarios to simulate
- Offering strategic recommendations to mitigate risks in conflicting scenarios
- Siloed information flow and forecasting practices
- Reactive response to changing market conditions, hyper-competitive landscapes, and evolving consumer preferences
While predictive analytics has been largely used by businesses to forecast demand with considerable accuracy, recent advancement in data science indicates the huge potential of Gen AI or LLMs to surpass traditional forecasting methods.
Bridging the demand planning gap with Gen AI
The era of gen AI is a transition towards more effective and widely accessible forecasting tools. Rather than replacing existing AI and ML models, gen AI is taking the demand planning and forecasting paradigm to the next level. There are certain areas where gen AI can prove to be more capable:
- Creating a dynamic picture of demand by analyzing multiple micro and macro-level indicators, offering an outside-in perspective.
- Augmenting predictive capabilities which allow planners to spend less time manually fine-tuning forecasts and focus on strategic decision-making and rapid responses to market shifts.
- Empowering planners with divergent thinking and bringing to fore broader scenarios and possibilities that may be unaccounted for.
- Enhancing ability to identify opportunities, analyze trends, mitigate risks, and develop more robust recommendations for better decision-making
Gen AI use-cases for demand planning
Use-cases | How Gen AI can help |
---|---|
Insights for decision support | Gen AI can accelerate operations and decision-making with a chatbot-like interface accessing a wide array of real-time datasets, both internal and external. In a demand planner’s routine, a Gen AI chatbot can provide insights into the impact of events across various supply chain nodes, enabling quick, informed decisions. |
Identifying baseline scenarios | Gen AI tools can assist in identifying baseline scenarios by analyzing historical data and recognizing patterns, providing a solid foundation for scenario planning. It can also help formulate scenarios that align with emerging trends, allowing for more accurate and forward-looking planning. |
Scenario prioritization | Gen AI can combine scenarios and help to assess their potential implications, enabling more comprehensive scenario planning and risk analysis. Planning teams can systematically refine parameters and filter out less feasible scenarios, to secure a prioritized set for simulation. |
Strategy generation | Gen AI tools helps to explore potential courses of action that might not have been considered otherwise, enriching the organization’s strategic decision-making. It can aid in evaluating various ideas and actions generated for different scenarios, providing valuable insights into the most promising strategies for sustainability. |
Prioritizing commercial drivers | Gen AI excels at analyzing extensive historical data to discern patterns and correlations previously elusive. By identifying key drivers like seasonal trends and emerging market shifts, companies can anticipate demand fluctuations with greater accuracy, minimizing inventory imbalances. These drivers can vary across functions, channels, and markets, offering a tailored approach to demand planning. |
Collaboration | Demand planning teams work with other functions like sales, marketing, and finance. Gen AI can serve as the connective tissue between these functions, ensuring seamless data sharing and uncovering both known and unknown business drivers. It facilitates cross-functional collaboration by providing insights and predictive alerts, ensuring alignment across departments, from logistics to sales and marketing, leading to more accurate forecasts. |
Sigmoid’s framework of Strategic Demand Planning
At Sigmoid, we believe the most accurate forecasts come from a balanced approach that combines human expertise with advanced technology. While algorithms excel at identifying patterns in data, human insight is essential for interpreting deep insights that might go unnoticed by technology. Our strategic demand planning framework follows a ‘smart-touch’ philosophy, integrating both human and technological strengths to deliver optimal, precise forecasts in a dynamic environment.
Our machine learning engine generates an unbiased, accurate baseline forecast using market intelligence and a deep understanding of demand drivers. The gen AI interaction layer enables automated enrichment to ensure this baseline forecast is enriched with relevant information to achieve the desired forecast accuracy.
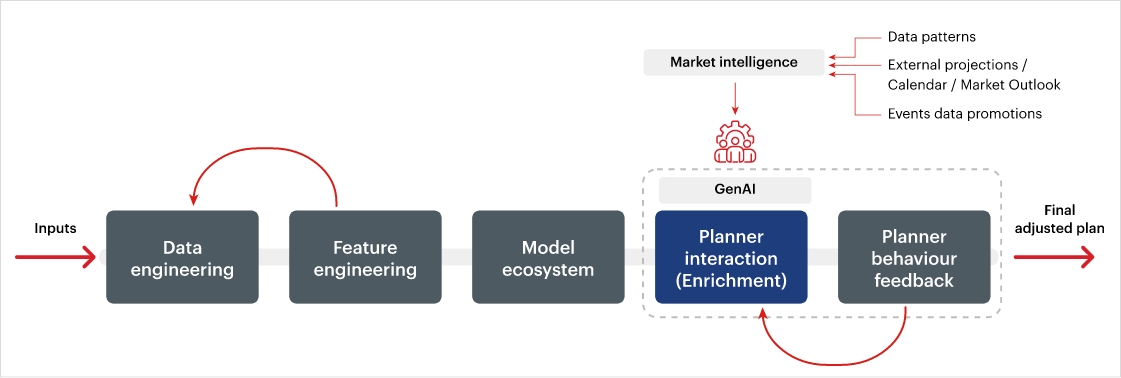
Gen AI can facilitate effective demand enrichment through the following capabilities:
- Synthesizing data business assumptions from various enterprise planning systems, business documents, unstructured datasets, and assumption repositories for future planning
- Unlocking market intelligence from business data by establishing relationships with measured data for a more holistic measurement of planning outcomes
- Incorporating diverse commercial inputs into the planning process, including sales data, promotion data, market trends, and consumer behavior insights and allocating weightage to these drivers for refining strategies for specific functions, channels, and markets
- Generating future scenarios and shortlisting them by assigning a high-medium-low likelihood of occurrence and determining outcomes for the prioritized demand scenarios
- Observing and indicating changes in data across time periods in the form of insights
- Optimizing forecasts based on historical changes and planner feedback
- Facilitating real-time calculations with granular insights for decision-making
- Establishing benchmark ranges for key metrics such as forecast accuracy and bias
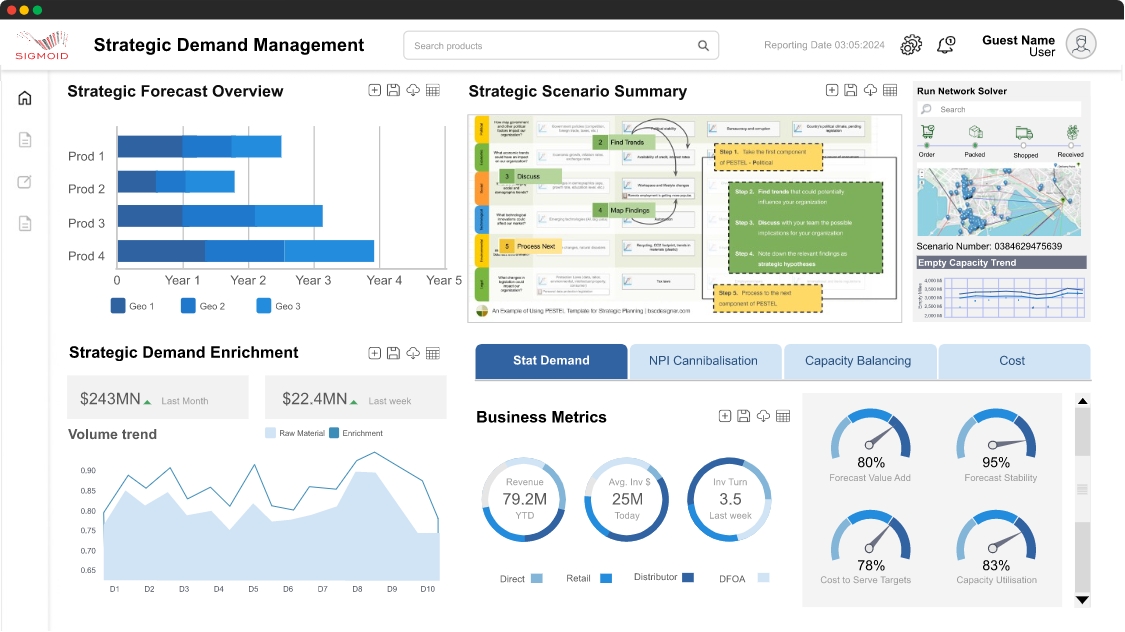
Fig 1. Gen-AI based strategic demand management tool (indicative dashboard)
Best practices for implementing Gen AI in demand planning
Before deploying and scaling Gen AI, it’s crucial to assess its feasibility, evaluate its true value, and determine its scalability within the organization to fully realize its benefits. Here are a few best practices for success in demand planning:
FAQs
- Demand Forecasting involves predicting future demand based on historical data, market trends, and statistical models. It typically looks at long-term trends.
- Demand Planning is a broader process that incorporates demand forecasting to align inventory, production, and resources to meet predicted demand while optimizing business operations.
- Demand Sensing is a short-term approach that uses real-time data, such as current sales and market conditions, to adjust forecasts and improve responsiveness to sudden demand shifts.
Demand planning is often conducted in the early stages of sales and operations planning (S&OP), a process for aligning supply with demand by coordinating sales planning and forecasting with production planning in a unified approach. Timely planning enables companies to make sure supply meets any expected demand fluctuations. It also informs the manufacturing process, while ensuring the capacity and availability of materials to meet projected production goals.
- Passive: The forecast incorporates historical and other data that the company collects, and projections are based on patterns and trends that have held up over time and may happen in the future.
- Active: The active approach takes into account aggressive growth plans such as marketing or product development and also the general competitive environment of the industry, including the economic outlook, market growth projections, and more.
- Short-term: Short-term demand forecasting looks at a small window of time to inform the day-to-day operations.
- Long-term: Long-term demand forecasting is conducted for a period greater than a year, which helps to identify and plan for seasonality, annual patterns, and production capacity.
- Internal (micro-level) forecasting: These forecasts use firm-level data including historical sales, past and current financial metrics and sales team projections, and customer data to predict demand for particular products and services.
- External (macro-level) forecasting: Macro-level demand forecasting is useful to incorporate larger industry-led, economic, national trends and other pervasive factors into an organization’s planning and projections.
- Demand Planning: Focuses on predicting future customer demand to ensure the right amount of inventory, resources, and production are available. It aims to align business operations with customer needs.
- Supply Planning: Concentrates on managing the production, procurement, and delivery of goods or services. It ensures that sufficient supply is available to meet demand while optimizing costs, lead times, and capacity constraints.
Many different methods can be used to forecast demand. While some businesses employ a simple single technique, other businesses may employ mixed-method approaches that combine large data collection efforts with machine learning techniques to determine forecasts. However, demand forecasting techniques may be broadly categorized into qualitative and quantitative approaches.
Qualitative demand forecasting methods include Delphi method, salesforce composite forecast, expert opinion, market research/surveys, focus group customer interviews. Quantitative demand forecasting methods include moving averages, trend projection and simple regressions, seasonal averages, Econometric modeling (advanced regressions), gradient boosting and other machine learning tools.
Featured blogs
Talk to our experts
Get the best ROI with Sigmoid’s services in data engineering and AI