Driving regulatory success in capital markets with data governance
Reading Time: 5 minutes
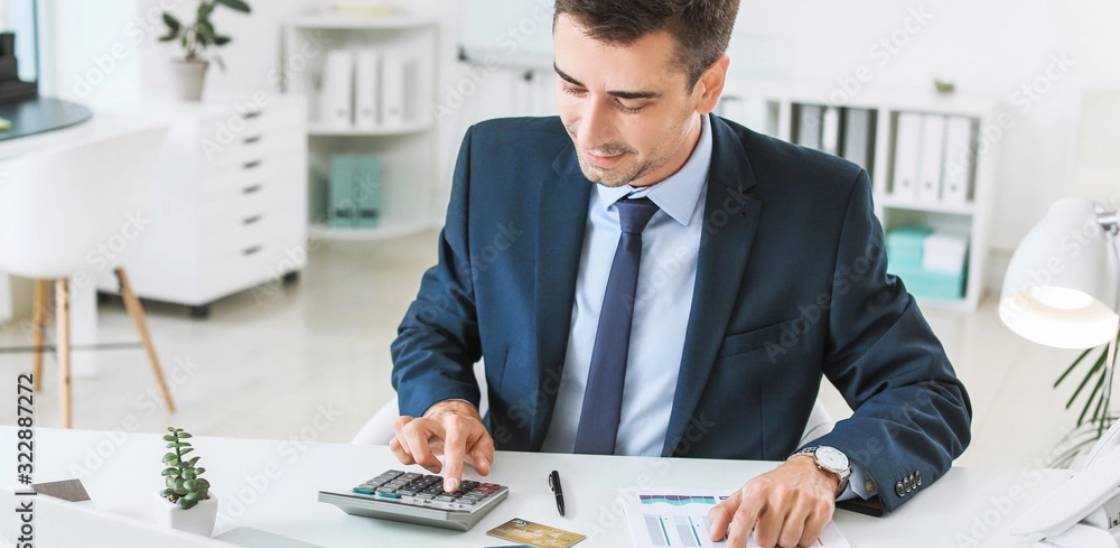
The ever-evolving regulatory landscape of capital markets feels like a tightrope walk. One misstep with data management, and brand reputation along with bottom line – could take a tumble. New rules and amendments are frequently introduced, such as the Securities and Exchange Commission’s (SEC) recent move to shorten the settlement cycle from T+2 to T+1. Under the new “T+1” settlement cycle, all applicable securities transactions from U.S. financial institutions will settle within one business day of their transaction date.
(read the complete SEC article here).
Keeping up with these evolving laws and changes heightens the risk of missing critical updates, which can lead to financial implications. In April, five registered investment advisers agreed to pay a total of $200,000 in penalties for allegedly violating the SEC’s amended marketing rule.1
Data governance emerges as a key solution to this problem, by implementing robust data governance practices, companies can create a framework that ensures data accuracy, consistency, and accessibility across the organization. It helps in maintaining up-to-date and reliable information, which is crucial for compliance with the latest regulatory changes.
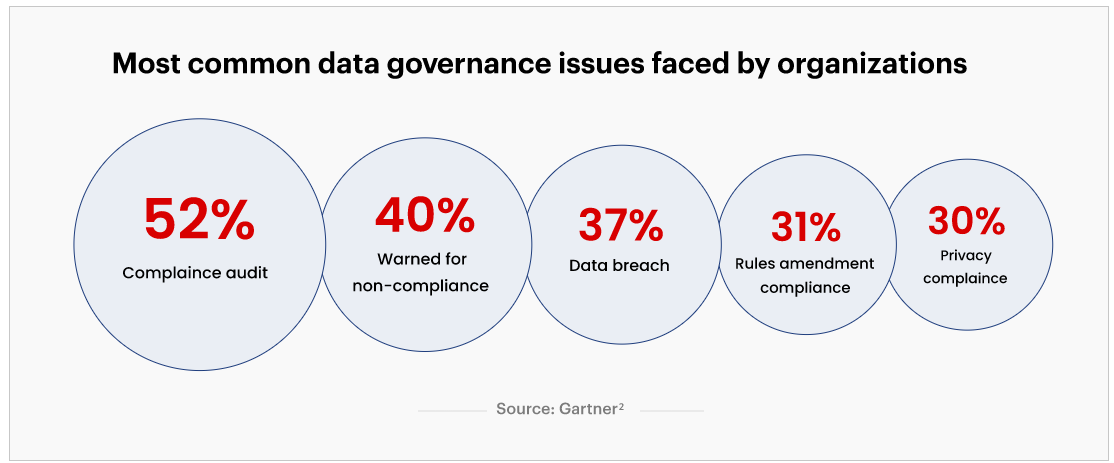
Understanding data challenges in regulatory compliance
Consider a scenario where a global investment bank operates across multiple regions, each with its regulatory nuances. The absence of standardized data formats and disparate management systems hinder the bank’s ability to aggregate and analyze data efficiently. Financial institutions encounter multifaceted challenges in regulatory compliance that demand nuanced solutions. Here’s a deeper dive into these hurdles:
- Fragmented data across disparate systems and departments hinder comprehensive
visibility and analysis. - Siloed data storage exacerbates the fragmentation issue, making it difficult to
aggregate information for regulatory reporting.
Lack of data standardization:
- Absence of standardized data formats and definitions complicates data management
and interoperability. - Without uniform standards, reconciling data from diverse sources becomes a tough
task, leading to inconsistencies and errors in reporting.
Traditional data governance methods:
- Legacy data governance methodologies often rely on manual processes and
spreadsheet-based tracking, which are prone to errors and inefficiencies. - These traditional methods struggle to keep pace with the volume, velocity, and
variety of data generated on a daily basis.
Key components of effective data governance
Adhering to best practices in data governance is not just advisable—it’s essential. These practices serve as guiding principles for organizations striving to maintain compliance, mitigate risks, and unlock the full potential of their data assets.
- Data quality management: Robust data quality management processes ensure that data is accurate, consistent, and reliable. Automated data profiling tools identify anomalies and inconsistencies, improving data accuracy. Leading platforms employ machine learning algorithms for automated anomaly detection, enhancing data reliability.
- Data lineage and traceability: Comprehensive solutions provide a clear audit trail, ensuring compliance with regulatory requirements. Blockchain technology offers immutable records, facilitating robust traceability. Clear tracing of data origin and transformation ensures transparency and accountability in data management.
- Data security and privacy: Advanced encryption, authentication, and masking technologies protect confidential data. Encryption algorithms such as AES secure sensitive financial information both at rest and in transit. State-of-the-art solutions leverage homomorphic encryption for secure computation without compromising privacy.
- Regulatory reporting and auditability: Automated systems streamline capturing, validating, and submitting regulatory reports, ensuring compliance with mandates. APIs and data integration aggregate data from disparate sources for accurate reporting. Consistent and accurate regulatory reporting meets obligations efficiently.
Data quality underpins effective data governance
Robust data quality management is essential for data governance because it enables organizations to trust and rely on their data for decision-making, analysis, and operational processes. Robust data quality management solutions generate diagnostic reports that provide insights into the overall health of data and flag any anomalies. This involves comprehensive data validation and profiling, with the ability to seamlessly integrate with various data sources such as flat files and databases.
Data quality is often assessed using six key dimensions:
- Accuracy: Ensures that data correctly represents the real-world entities or events it is intended to model.
- Completeness: Measures whether all required data is available and collected.
- Consistency: Ensures that data is consistent across different data stores and formats.
- Timeliness: Refers to whether data is up-to-date and available when needed.
- Validity: Checks if data conforms to the required format, type, and range.
- Uniqueness: Ensures that each data record is unique and there are no duplicates.
Sigmoid offers a comprehensive data quality management platform that operates based on these governing principles. This plug-and-play solution has been successfully implemented in large-scale data quality management projects, demonstrating its capability to enhance data health and integrate seamlessly with existing workflows.
Case in point
Explore how Sigmoid’s innovative solutions can transform risk management, as demonstrated in the case study below.
Situation | Solution | Impact |
---|---|---|
A global investment bank sought to enhance its data infrastructure to comply with regulations such as MAR and MiFID. Their goal was to establish robust data pipelines, real-time data mapping, and a customized in-house tool to ensure regulatory standards were met. | Sigmoid developed a detailed alerting workflow and interface, enabling tuning and iteration of the trade surveillance model based on compliance team requirements. The custom-built architecture allowed them to classify fraudulent transactions and implement surveillance. |
|
The role of generative AI in data governance
The advent of generative AI represents a paradigm shift in data governance. This groundbreaking technology automates mundane tasks, augments human capabilities, and enhances overall efficiency. A regulatory compliance team spends countless hours manually documenting data sources and attributes. By leveraging generative AI, the compliance team, along with data management, analytics, or risk management teams can automate this process, freeing up valuable time to focus on higher-value tasks such as regulatory analysis and risk assessment.
Generative AI also offers capabilities in synthetic data generation, enabling organizations to create realistic yet anonymized datasets for testing and training needs. In the context of regulatory reporting, synthetic data can be used to validate reporting algorithms without exposing sensitive information.
Investment banks can also leverage generative AI to process vast amounts of information from compliance documents. It can efficiently analyze and compare different versions of documents, quickly identifying changes and updates. This capability is valuable for maintaining up-to-date compliance records and ensuring that all data governance practices adhere to the latest regulatory requirements.
Conclusion
In capital markets, compliance is non-negotiable and the pursuit of effective governance is a strategic imperative driven by data. As we look at the critical role of data governance in preserving trust and credibility, companies must realize that its true value lies in driving operational excellence and fostering a culture of accountability and innovation. Financial institutions today are putting themselves in a position for long-term success by placing a high priority on strong data governance.
For a more in-depth exploration of leveraging data and analytics to streamline regulatory compliance management in the banking and financial services industry, read our whitepaper: Regulatory compliance management for banking and financial institutions: The data and analytics way
Sources:
Featured blogs
Subscribe to get latest insights
Talk to our experts
Get the best ROI with Sigmoid’s services in data engineering and AI
Featured blogs
Talk to our experts
Get the best ROI with Sigmoid’s services in data engineering and AI