Cloud data modernization for business growth: Insights from industry leaders
Reading Time: 6 minutes
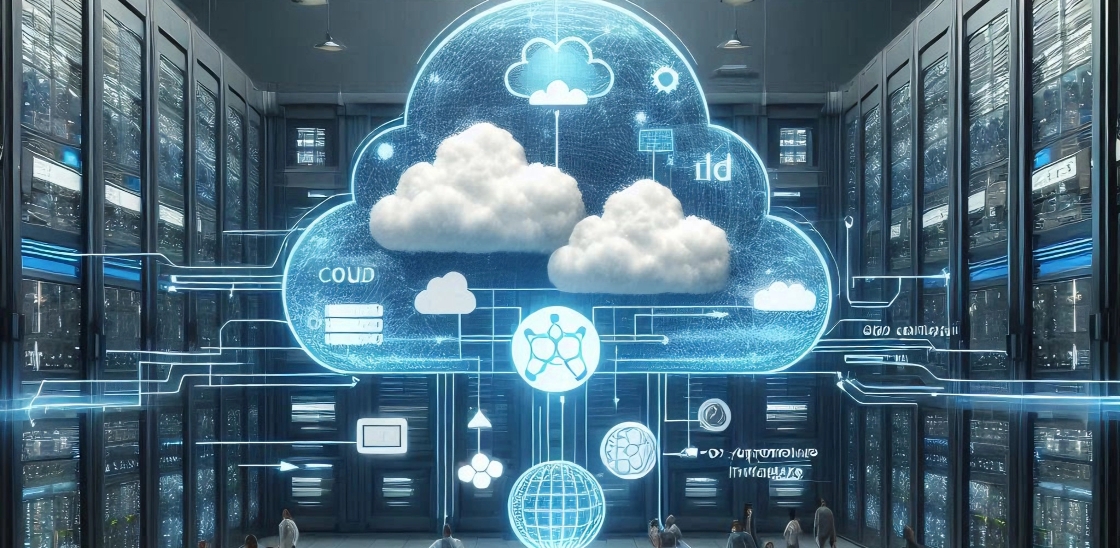
According to the 2023 Cloud Modernization Research Report by AWS, 80% of IT decision-makers agree that failure to modernize legacy applications and data solutions will prevent them from fully leveraging AI.
Cloud adoption is driven by the need for scalable, flexible infrastructure that can support technologies like AI. However, as businesses embark on their modernization journey, they face a complex web of challenges, from migrating legacy systems to optimizing costs and ensuring data security.
In our latest webinar on “Cloud Data Modernization as a Catalyst for Business Growth and Innovation,” Sigmoid’s CTO Mayur Rustagi discussed the impact of macroeconomic factors, challenges of legacy system migration, and the role of AI in shaping the future of cloud computing with the Global Head of Data Platforms & Engineering of a leading global business data and analytics company. This blog delves into key insights on how cloud data modernization is driving business growth.
Q1. In what ways are macroeconomic factors impacting cloud spending decisions for businesses?
A. Despite challenges in the macroeconomic environment—such as inflation, geopolitical unrest, and ongoing conflicts—companies are continuing to invest in cloud technologies. While the overall outlook may seem negative, businesses are strategically spending in crucial areas to maintain a competitive edge.
- We observe significant investments in AI, generative AI, and cloud infrastructure to drive innovation and efficiency.
- There is a heightened focus on optimizing spending across infrastructure, compute, storage, and software to reduce costs.
- Despite the economic challenges, cloud spending remains resilient, with public cloud providers reporting positive growth quarter over quarter.
Q2. What are your views on cloud modernization and how do you manage governance, security, and costs during cloud data modernization processes?
A. Our focus has been twofold: One, modernizing our legacy applications and infrastructure, and building new capabilities. We are working to move away from our mainframe systems and consolidate some of our legacy applications into more modern, efficient solutions. Two, we’re simultaneously investing in new capabilities, particularly in our data and analytics platform. To manage governance, security, and costs during this cloud data modernization, we implement strict compliance measures, robust security protocols, and continuous cost monitoring to ensure an efficient and secure transition.
Q3. What specific challenges have you encountered in migrating away from mainframe systems? How does this compare to the difficulty of migrating from other legacy systems or data warehouses?
A. One of the biggest hurdles is dealing with legacy code, such as COBOL or JCL, which often consists of thousands of lines. Understanding and working with this code can be difficult, especially since many people who originally wrote it may no longer be with the organization. Additionally, there aren’t many plug-and-play solutions in the market that allow a straightforward migration of code and data.
Being a data-centric company, when we migrate legacy code—whether from mainframes or other older systems—into newer technologies, it involves extensive data testing. This is crucial because our data is integral to many products that generate revenue and are used by major banks and financial institutions to make critical decisions. We must ensure that the data and functionality remain intact, which requires thorough testing and validation.
Q4. How do you manage business expectations and ensure timely delivery during the migration process?
A. Businesses face the challenge of balancing immediate business needs with long-term technological advancement. This dilemma often manifests into a struggle between maintaining legacy systems and investing in new, revenue-driving capabilities. To navigate this complexity, they should consider the following key points:
- Strategic balance: Strive for equilibrium between maintaining the legacy footprint and building new capabilities. Both aspects are crucial for sustainable growth and require careful resource allocation.
- Adaptive long-term vision: Develop a clear, long-term strategy and roadmap while maintaining the flexibility to adapt to short-term challenges.
- Senior-level alignment: Ensure that key stakeholders, including the Chief Product Officer, Chief Technology Officer, and leaders from product, business, tech, and sales teams, are unified in their understanding and support of the strategy.
Q5. How do you manage the challenge of balancing internal teams that know your legacy systems with external cloud experts who lack that familiarity?
A. When updating a company’s technology, it’s crucial to balance the knowledge of existing teams with the expertise of external specialists. This challenge is common in many businesses undergoing digital transformation. Here’s how we can approach this balancing act:
- Develop internal talent: Prioritize hiring skilled professionals and invest in their growth through training and mentoring. This approach builds a strong foundation of in-house expertise.
- Establish clear objectives: Set well-defined goals and timelines for the next one to three years. This clarity can help align efforts and make the transformation more manageable.
- Leverage external partnerships: Recognize internal limitations and collaborate with specialized external partners to fill skill gaps. It ensures the right mix of institutional knowledge and new expertise for each project.
Q6. Question to Mayur: As a services partner working with large companies, what has been your experience with cloud adoption and the shift to AI?
A. We help companies as a guiding partner to navigate the complex journey of cloud adoption and AI integration, touching on every part of their IT ecosystem. With experience dating back to 2013-2014, including early installations of platforms like Snowflake and Databricks, we bring deep expertise in cloud migrations. Our approach involves developing tailored strategies, guiding decisions between “lift and shift” or transformative overhauls, and leveraging proven patterns for migration. We also provide accelerators that streamline the process, such as tools for cloud monitoring to optimize cloud costs and resource utilization. Ultimately, our goal is to support clients with the right strategies, tools, and talent for successful cloud and AI transformations.
Q7. Question to Mayur: Everybody wants to get into the AI arena, so tell me a little bit about what you have seen as the bottlenecks for AI adoption and AI implementations.
A. As artificial intelligence continues to expand its transformative capability, businesses are eager to harness its potential across use cases. However, the path to successful AI adoption is not without obstacles. Two major challenges stand out in the implementation of AI systems:
- Data quality and management: AI models, especially in areas like Generative AI, require vast amounts of high-quality, relevant data. Organizations often struggle to create and maintain the robust data ecosystems necessary to feed these hungry algorithms. Without proper data infrastructure, companies risk inefficiencies and inflated costs in their AI initiatives.
- Scaling from development to production: While many AI projects show promise in controlled environments, they face significant hurdles when deployed at scale. In real-world applications, these models need constant monitoring for performance, data drift, and accuracy. Failure to maintain model quality can lead to incorrect outputs, potentially eroding trust among business leaders who rely on AI-driven insights.
Q8. Can you share some key insights from your company’s experience with AI implementation?
A. Our AI journey centers on robust data management. We’ve established a dedicated Data Supply Chain team responsible for processing, cleansing, and maintaining high-quality data from various internal and external sources. This forms the foundation for our AI projects, including revenue-generating applications, AI-based co-pilots, and partnerships with large clients to develop AI solutions.
We face challenges in integrating diverse data sets, particularly as we work across different sectors like financial services and consumer goods. Each industry has its own unique requirements and considerations, making data integration complex. Additionally, given the sensitive nature of the information we handle, a key focus is addressing data privacy, compliance, and security concerns as we expand our AI capabilities.
Q9. How do you track and optimize resources after migrating to a new cloud platform? Do you use any specific tools?
A. Establishing a strong foundation of governance and observability is essential. This includes implementing a solid governance framework for tagging projects, managing leases, and overseeing infrastructure. It’s important to set up alerts and maintain end-to-end observability across all layers. Once foundational governance is in place, tracking and optimizing costs can include:
- Categorization and tagging: Use consistent tagging practices to categorize projects and resources to track costs associated with specific projects or departments.
- Cost management tools: Leverage built-in tools from cloud providers such as AWS Cost Explorer or Google Cloud’s cost management features to monitor and analyze spending.
- Cost controls: Set up alerts for cost overruns and automate actions like shutting down unused instances during weekends or holidays.
- Regular reviews: Continuously review and adjust your cost management strategies based on usage patterns and performance metrics.
Conclusion
As businesses navigate the complexities of cloud data modernization, success hinges on balancing existing expertise with the adoption of cutting-edge technologies. By prioritizing strategic planning, robust data management, and continuous optimization, organizations can fully unlock the transformative potential of cloud technologies and AI.
Featured blogs
Subscribe to get latest insights
Talk to our experts
Get the best ROI with Sigmoid’s services in data engineering and AI
Featured blogs
Talk to our experts
Get the best ROI with Sigmoid’s services in data engineering and AI